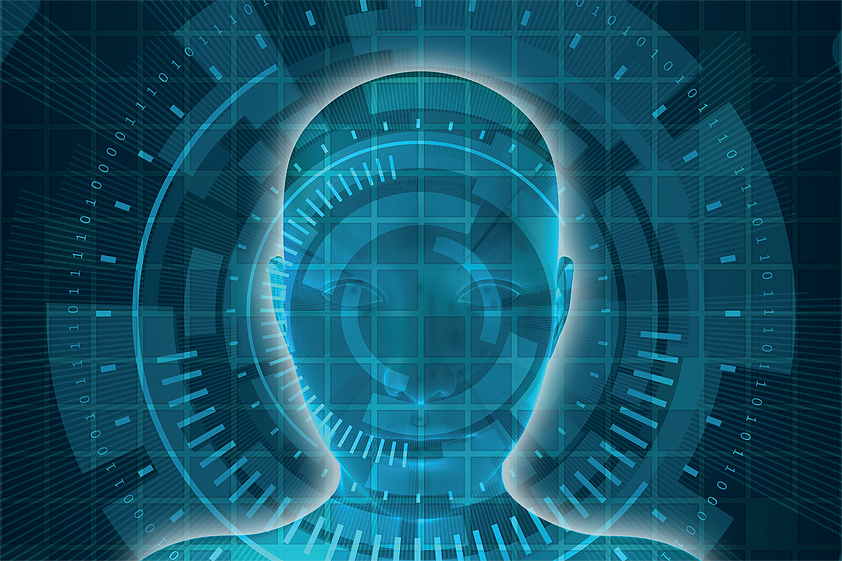
The Lights Are On: Why Augmenting Human Planners with AI is the Best Path Forward for Supply Chains
The world-renowned physicist Stephen Hawking warned that artificial intelligence could replace humans, and with AI adoption increasing and the idea of a ‘lights out’ autonomous supply chain looking possible, it’s high time to consider if this is really the best route forward.
AI, including its most used algorithms of machine learning (henceforth AI/ML for short), holds incredible promise for supply chains. AI/ML and the broader field of data science which I sometimes call ‘fancy maths,’ comprise an amazing pattern-finding machine. But the power of these patterns for a supply chain has its limits. The best way to have an impact is not to turn off the lights and send the people home but to augment your planners with AI/ML so each is at its highest and best use.
AI/ML adoption is on the increase, and the pandemic has accelerated this trend. Fifty-two percent of executives say AI tools have boosted productivity, PwC AI Predictions, 2021, and global analyst Gartner predicts that 75 per cent of organizations will move from piloting to operationalizing AI by 2024. A recent IBM survey found its ranking has risen on the transformation agenda from fifth to second. The question is not if companies will adopt AI/ML but how they should do so.
In general, AI/ML algorithms are masters at finding patterns in large amounts of data, but the key word here is ‘large.’ Since the maths ‘learns’ by looking for patterns, it most commonly is ‘trained’ using copious amounts of historical data. Once it has learned the pattern from the training data, the algorithm uses that pattern to make predictions on an entirely new data set. These AI/ML algorithms themselves are often (although not always) combined with a set of processing rules to automate a response to the prediction. These machine insights and automation can tackle a scale far beyond the cognitive capacity of humans.
This pattern-finding ability can ‘sense’ demand from signals beyond traditional sales data, thus offering enhanced sources to improve forecast accuracy. It can predict what lead times will actually be based on history instead of human guesswork, saving a planner from tedious manual analysis of a complicated bill of materials. It can forecast and optimize trade promotions. These are but a few examples of applications that could aid supply chains. After all, striving to build a better plan is a perennial goal of supply chains, and increasing plan accuracy with algorithms is making an impact.
But while AI research has advanced by leaps and bounds these days, accuracy must be kept in equilibrium with agility. All perfect plans are upended by supply chain disruptions, and the times are growing more, not less volatile. The ability to respond to these events quickly is an equally important muscle to build. We want the best insights we can build to increase accuracy in an agile supply chain run by humans. Because humans are still better than machines in some core areas, which is why the lights aren’t going out any time soon. I’ll highlight three capabilities where people excel: context, collaboration, and conscience.
When AI/ML learns from historical patterns in large volumes of data, it can be confounded when confronted with volatile data and especially with unprecedented events. Supply chain disruptions, particularly those with the magnitude of the pandemic, make pattern-finding a challenge, and algorithms are unable to understand what is happening. Humans uniquely possess the ability to derive meaning from context, so when disruptions disrupt the models, it is planners who are able to use business acumen and domain expertise to make the best decisions.
For example, during the pandemic, forecasting models powered by machine learning couldn’t sense demand no one could understand, so in the interim planners had to use their domain expertise and context to made the best decisions possible. After a few weeks of sales, a team of data scientists at Kinaxis were able to apply other predictive modeling techniques not reliant on finding patterns in long-term sales history to find clusters in the most recent sales data, which they then used as a rougher forecast estimate. The combination of humans understanding the context, making initial decisions, and then guiding algorithms for short-term approximations rendered better decisions.
Similarly, AI/ML can find patterns in data relationships, but algorithms do not understand relationships between people. Supply chains may be a process for making and moving stuff but underlying the links in the chain are relationships between people. The pandemic exposed the criticality of these relationships, as companies found collaboration was one of the most essential components to managing the disruptions their company experienced. This collaboration took many forms.
Colleagues and internal functions worked together in new ways, as everyone pitched in to mitigate the crisis. Companies worked closely with their suppliers, who faced their own challenges, to ensure survival of these smaller companies upon which their supply chain depended. And non-traditional partners emerged as companies collaborated with those outside their industry to manufacture products like personal protective equipment, sanitizer, and ventilators, or provide services like assistance with transporting products.
Finally, AI/ML is a bundle of algorithms but has no conscience. It is up to humans to guide and determine whether the fancy maths is used for good or for ill. There are ample examples at both ends of the spectrum. While the United States and China as individual countries have led research advances in AI, the European Union has argued that as a collective the continent is best positioned to lead in advocating for ‘human-centric’ AI that is trustworthy and in keeping with European values. Interest in protecting privacy is higher in EMEA, which led to European leadership in establishing General Data Protection Regulation (GDPR), so the same vision can inform AI/ML.
One key element in building trust with AI/ML is interpretability. While these models are usually famously black box in nature, there are tools that exist to shine light into the box and help the planner understand the factors that drove the prediction. One such approach is SHAP (SHapley Additive exPlanations), which can be used in all kinds of ways, such as showing the features that most impacted a ML-powered forecast in demand sensing. As planners can test and see the factors impacting their forecast they are more likely to adopt AI/ML tools. In fact, GPDR includes a set of rights related to the explainability of AI.
Another factor central to building trust in AI is expanding its access beyond the narrow cadre of data scientists. Fortunately, an emerging approach called automated machine learning (or AutoML) is expanding access to these techniques to whole new categories of people without requiring rarefied expertise. By automating many of the steps involved in building a machine learning pipeline, a business user without training as a data scientist can build many usable models, especially for areas like common forecasting tasks. This development is so promising that McKinsey predicts demand for what they call AutoML practitioners to be double that of data scientists. When AutoML is paired with tools to ensure interpretability of models to mitigate their black box nature, the combination can ease adoption and offer tremendous business value to a far wider range of companies, including their supply chains.
Trust in the fancy maths is critical, but so is transparency in the data. People all along the entire supply chain, from the planners of materials, production, demand, inventory, etc. need to have visibility into the same data at the same time. It’s called a supply chain because the functions are linked, which means each impact the other. Cascading information down the chain means waiting for someone’s updates to be entered and creates latency that can lead to finger-pointing and erode trust. The impact of agility and the insights rendered by both humans and machines are limited if transparency is lacking.
To prevent planners from being mired in tedium, AI/ML can use machine intelligence to automate mundane tasks and deliver insights that humans just can’t see. This opens up a world where planners can apply their capabilities of context, collaboration, and conscience to focus on exceptions and manage the complexity beyond the scope of machines. By putting the power of the complex maths into the hands of far more humans by using AutoML, supply chains can far more effectively scale application of AI/ML. These people will adopt the AI/ML if it includes the tools to insure its interpretability.
Planners can know what’s happening sooner and act faster on that information if they have a foundation of agility and transparency. People and algorithms work best together, with the lights on, so for supply chains the best direction is to augment people power, rather than replacing it.
Polly Michell-Guthrie is VP Industry Outreach, Kinaxis. Everyday volatility and uncertainty demand quick action. Kinaxis® delivers the agility to make fast, confident decisions across integrated business planning and the digital supply chain. People can plan better, live better and change the world. Trusted by innovative brands, Kinaxis combines human intelligence with AI and concurrent planning to help companies plan for any future, monitor risks and opportunities and respond at the pace of change. Powered by an extensible, cloud-based platform, Kinaxis delivers industry-proven applications so everyone can know sooner, act faster and remove waste.
www.kinaxis.com/en