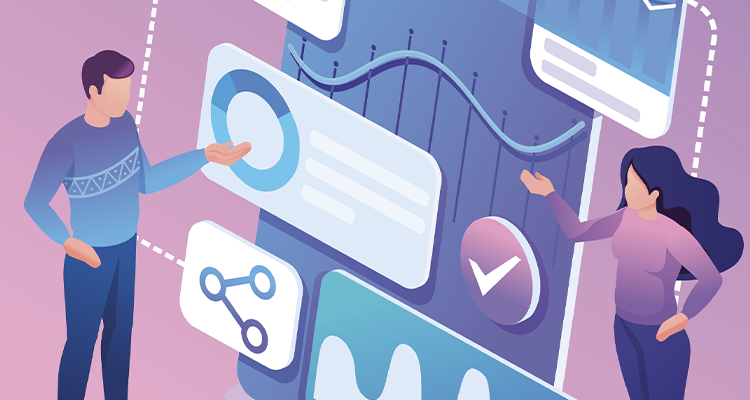
Transplant connective tissue into your supply chain for greater agility By Mark Holmes
Adapting fast to events and trends such as energy-price shocks, production shortages, or sudden changes in consumer demand, has become a critical capability for supply chain organizations. Our world is far more volatile, and organizational success requires greater levels of agility than ever before.
It is about more than minimizing disruption – it is about the ability to adapt faster than the competition to optimize the supply chain more intelligently and consistently to maintain resilience and increase revenues.
The problem for most large organizations now is they have increasingly complex supply chains. Many organizations have 20 or 30 applications covering the management of transportation, orders, warehouse operations, and yard movements at manufacturing plants or depots. Increased agility requires the ability to connect enterprise applications and gain a single source of truth from their disparate data, which is very difficult without a purpose-designed connective tissue to harmonize it all and make it fit for analytics.
Supply chain digital maturity
The reality for most organizations is that a faster, more dynamic supply chain requires a certain level of digital maturity that is much farther on from where they are.
If the first level is about foundational architecture and connectivity, the second level of maturity is where an organization has achieved end-to-end visibility, automation, and smart asset management. The third level is where greatest agility is achieved through access to predictive capabilities and the introduction of automation into decision-making. It enables greater collaboration and integrated planning and adaptive orchestration, preferably through a control tower.
Fully optimizing a supply chain at this level, with near-real-time predictive and prescriptive insights demands AI and machine learning (ML). This is how organizations accelerate the speed and quality of decision-making at critical moments. Analysts at Gartner expect that by 2026, 50 percent of supply chain organizations will use ML to augment decision-making.
If, for example, a supplier cannot meet production requirements for a new order, the supply chain organization needs to find alternatives fast. Even better – it should have advance warning of such problems which may be caused by a shortage of raw materials further back in the chain. Organizations need analytics-powered real-time demand sensing and forecasting capabilities to keep ahead of such events. And once they have access to prescriptive insights, they benefit from recommendations on the best options they have to fulfill an order and ensure optimum ROI.
It may be, for example, that there is sufficient inventory in transit or in warehouses that can be brought together to meet a surge in demand without the cost and disruption of engaging a new supplier or activating extra manufacturing capacity. Organizations can only know this through near-real-time visibility of every element of their supply chain.
The challenges of disparate data
What stands between so many companies and these advanced orchestration and fulfillment capabilities are the problems of normalizing many sources and types of data. In most cases this is impossible without a new connective tissue to bring it all together harmoniously. Only then can companies move to the third level of digital maturity that enables them to use AI and ML.
Companies are increasingly aware they need these capabilities and that they must provide a bridge between applications and data silos. In IDC’s 2022 Supply Chain Spotlight Survey, when participants were asked about the steps their companies are taking to mitigate their supply chain planning challenges, 34 percent said they are looking for ways to better integrate their supply chain applications. They know they must integrate their supply chain data more effectively so they can use insights to make intelligent, real-time decisions at pivotal moments.
It is not another application that supply chain organizations need but a single source of truth from all their disparate data and the ability to sense and forecast demand and optimize fulfillment as constraints change. Organizations need to harmonize disparate data and make it easy for themselves to use AI and ML.
The data fabric
In the real world, this is only possible through adoption of a data fabric approach – an architectural approach founded on a unified data platform that has embedded AI and ML capabilities. This should be able to ingest data from any source and in any format, including real-time event and transactional data as well as data at rest. Such a platform will integrate data and processes from different systems for intelligent, informed decision-making.
Taking this one step further is the smart data fabric approach. This relies on a data platform with embedded analytics provides line-of-business decision-makers with access to descriptive, predictive, and prescriptive insights that transform their ability to make the right calls when the pressure is on. They can see what is likely to be a problem or opportunity so early they achieve vastly better outcomes than by relying on any other approach.
The ability to adapt models to incorporate the most relevant information, from a range of disparate sources and to access AI and ML capabilities, is critical for organizations wishing to stay one step ahead of the competition. This is especially true in an unpredictable world where experience alone is not enough to foresee and deal effectively with every threat and opportunity.
For a list of the sources used in this article, please contact the editor.
Mark Holmes
Mark Holmes is Senior Advisor for Global Supply Chain, at InterSystems. Established in 1978, InterSystems is the leading provider of next-generation solutions for enterprise digital transformations in the healthcare, finance, manufacturing, and supply chain sectors. Headquartered in Cambridge, Massachusetts, InterSystems cloud-first data platforms solve interoperability, speed, and scalability problems for large organizations around the globe.